6 min read
From Noise to Signal
A closed-loop data model for portfolio optimization.
Key Takeaways
Actionable product demand and usage feedback must find its way back to portfolio managers for continuous portfolio optimization to occur.
Morningstar provides a framework and measurement for matching clients’ risk tolerance with portfolio risk.
Morningstar has introduced several tools to support the risk-matching model, including the Morningstar Portfolio Risk Score and the Morningstar Risk Profiler.
A differentiating attribute of software companies is the way they integrate customer feedback into product development. Software is designed and built so that its use creates actionable data, which is fed back into product development as a closed-loop process of continuous improvement. The shorter the learning loop, the faster the actionable data improves the software. Think of Tesla’s use of driver data to develop autopilot, or Amazon’s use of digital analytics to improve eCommerce.
Asset and wealth management are also software-intensive industries. Yet it can sometimes be hard to identify the workings of a closed-loop process that optimizes the financial product (an investment portfolio) based on data collected from the customer (an institutional or retail investor). What actionable data is needed to close the portfolio optimization loop?
Closing the Loop
Unlike in direct-to-consumer businesses, asset and wealth management have long and complex value chains. These value chains connect heterogeneous manufacturers of financial products with a variety of distribution channels (some owned, some independent) and customer endpoints.
From a data perspective, the challenge is manifold. First, the product itself has become more complex. Today’s asset managers increasingly sell portfolio solutions constructed from many different product parts. Since Morningstar launched its model portfolio database in 2019, over 2,500 models have been reported, with most of them designed to serve as the core of an investor’s portfolio by targeting a specific level of risk.
Demand and usage signals from investors must find their way back to portfolio managers across layers of intermediaries, systems, and communications channels. Signals must be extracted from the noise and made actionable: based on feedback, what portfolio optimization is required? Taken as a whole, the system requires data that achieves the right coverage (of investable assets), integration (into a portfolio view of risk), and enrichment (to distill an actionable signal).
A Framework for Matching Risk
How do we model these requirements? Start with the asset manager, whose primary job is to build portfolio solutions which match an investor’s need for exposure to a specific level of risk with an appropriately risky portfolio. To achieve this, we need a method for evaluating the investor’s risk tolerance, a method for evaluating the riskiness of a diverse portfolio of investment products, and a method for bringing them both together.
On one side of the equation, we can evaluate the client using a blend of subjective and objective risk factors (see chart). Subjective factors include the client’s risk tolerance (their psychological appetite for taking financial risks as expressed by, for example, their willingness to accept losses for a chance to make bigger returns) and their loss tolerance (how they feel and act when unfavorable outcomes occur). Objective factors include the client’s experience and knowledge, and the financial needs, liquidity, and time horizon for each investment portfolio.
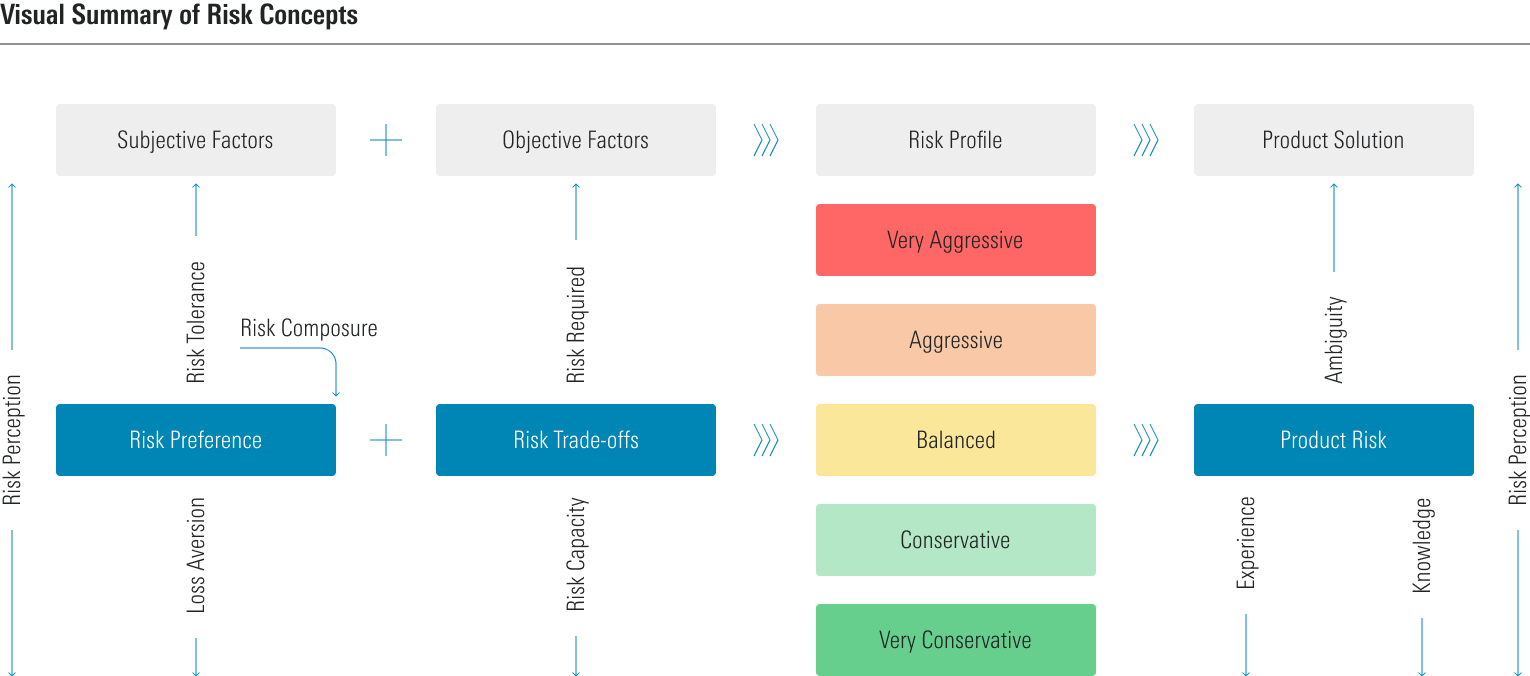
On the other side of the equation, we must evaluate product risk. In the middle, we calculate client and portfolio risk using a shared measurement and profiling system and match them together.
Our Tools
To support this risk-matching model, we’ve introduced several tools. For portfolio risk evaluation, we’ve built Morningstar Portfolio Risk Score (MPRS). Unlike portfolio allocation-based measures of risk, our tool uses a 30+ factor risk model to perform a robust assessment of volatility and correlations at the underlying holding level. The tool then benchmarks the portfolio against Morningstar’s Target Allocation Index family and assigns it a risk score of between 1-100, distilling thousands of data points into a singular and simple measure of risk.
For evaluation of the client’s risk tolerance, we’ve introduced Morningstar Risk Profiler. Based on a psychometric questionnaire originally developed in the 1990s by Finametrica, whose parent company we acquired in 2020, the tool scores the client’s risk comfort as a range of portfolio risk, using the same 1-100 scale. Once the financial advisor has adjusted the target level of investment risk for objective factors like client experience and portfolio time horizons, they can compare the client’s risk comfort level with the riskiness of their investment portfolios and make further refinements as needed.
We have more work to do. MPRS does an excellent job with vanilla investments like stocks, bonds, and cash. We must now push deeper into alternative investments like crypto assets, annuities, structured products, and private equity. Increasingly, financial advisors are recommending more complex products. And even if an advisor would never recommend Bitcoin ETFs for a retirement portfolio, many clients will walk through their door holding these assets.
Any closed-loop process will also continue to rely heavily on human intermediation—for example, between financial advisors and their clients, or between financial advisors and their home offices. But the simplicity of a singular risk score has the potential to bring data integrity even to complex human-machine systems like this. Our hope is that it becomes a lingua franca for portfolio optimization that ties client, financial advisor, asset manager and investment portfolio together, to the mutual benefit of all.